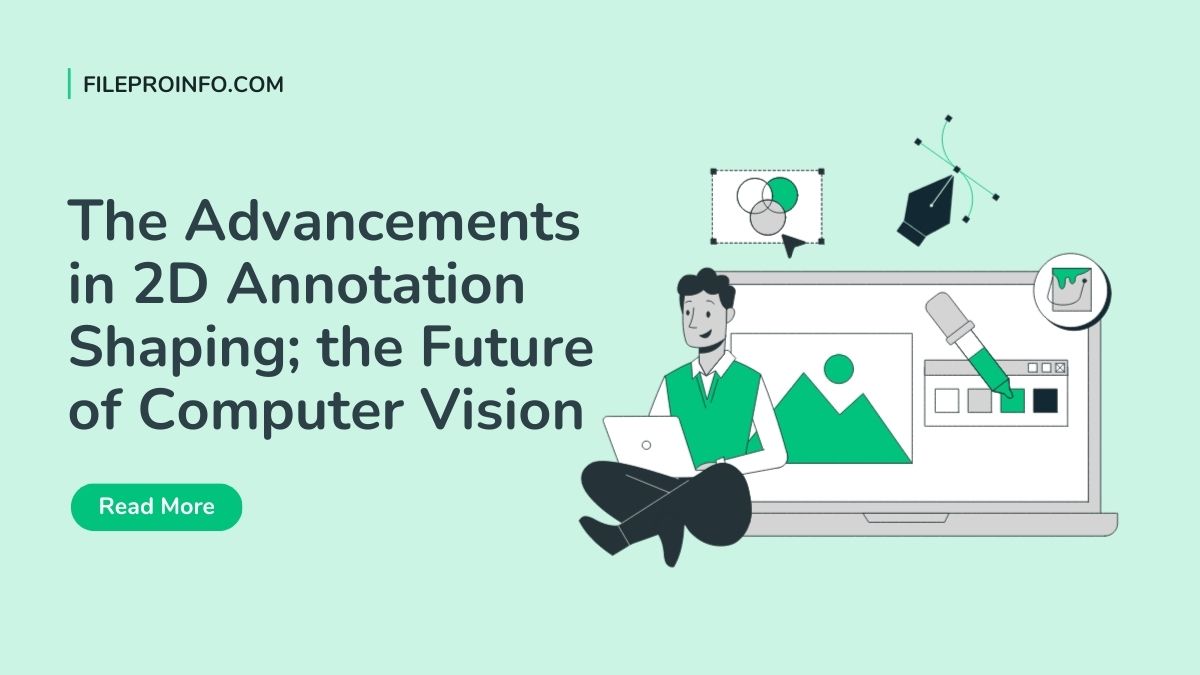
Computer vision, which deals with enabling computers to understand and interpret data, has made progress in recent times. An essential aspect of computer vision is image annotation, where experts label objects in images to train machine learning algorithms. In the realm of 2D annotation, several innovative developments are influencing the future of computer vision.
1. Introduction to 2D Annotation
In 2D image annotation solutions, objects in images are labeled using bounding boxes, polygons, key points, or semantic segmentation masks to provide training data for computer vision models. This process aids computers in identifying and recognizing objects. With advancements in deep learning algorithms and hardware capabilities, training computer vision models have become more accessible. This has resulted in a higher demand for top-quality annotated datasets.
2. Automation in 2D Annotation
Traditionally annotating images using 2D techniques has been a time-consuming task that requires labelling by human experts. However, there is an emergence of automation techniques that are making the annotation process efficient. One such method is semi-learning, where algorithms assist annotators by suggesting potential annotations based on previous annotations. This approach reduces effort while enhancing consistency in annotations.
Another significant advancement in automation is the utilization of data generation. Scientists can now generate computer-generated images, which allows for the creation of annotated datasets. This approach reduces the need for time-consuming annotation. Synthetic datasets have the advantage of providing defined ground truth, enabling better model evaluation and benchmarking.
3. Active Learning and Intelligent Data Selection
Traditionally, annotating datasets required annotators to label thousands or even millions of images—an overwhelming task. Active learning algorithms aim to tackle this challenge by selecting informative samples for annotation, thereby significantly reducing the annotation workload.
Active learning algorithms prioritize annotating instances that prove challenging for the model by evaluating its uncertainty or confidence in its predictions. This iterative process enhances the model’s performance by focusing on cases and gradually decreasing uncertainty. Through learning, annotation efforts can be optimized resulting in precise and efficient training datasets.
4. Crowd Annotation and Crowdsourcing
There has been a rising trend towards using crowd annotation and crowdsourcing platforms to meet the increasing demand for data. These platforms leverage intelligence to annotate amounts of data quickly while keeping costs lower. By dividing the annotation tasks among annotators, the process becomes more scalable and efficient.
Platforms that involve crowd annotation also incorporate quality control measures, like majority voting and adjudication to ensure consistent annotations. These platforms promote collaboration among annotators. Enables feedback loops to enhance annotation quality over time.
5. Outsourcing and Annotation as a Service
To meet the increasing demand for datasets, many companies are opting for outsourcing and utilizing annotation as a service provider. These specialized providers offer annotation services, saving companies the time and effort required to assemble their annotation teams and infrastructure.
Outsourcing annotation tasks to experts allows companies to focus on their core competencies while ensuring top-notch image annotation services. Annotation service providers usually have a pool of annotators in various domains, ensuring precise annotations across different application areas.
6. Challenges and Ethical Considerations
Despite the progress in 2D annotation, there are still challenges that need attention. A primary challenge lies in ensuring diversity and inclusion during the annotation process. Due to its nature, biases can inadvertently creep in. Having a group of annotators is crucial to minimize bias and ensure representation.
Another challenge revolves around privacy and consent considerations. The images being labeled may contain personal information. It is vital to obtain proper consent from individuals and ensure the privacy of their data. Annotation workflows must follow guidelines and regulations to safeguard individuals’ rights and maintain trust.
Conclusion
The progress of computer vision heavily depends on advancements in 2D annotation. Techniques like automation, learning, and crowd annotation platforms are revolutionizing the process of annotation, resulting in enhanced efficiency and accuracy. Companies seeking datasets can benefit from solutions offered by outsourcing or annotation as a service provider.
As the field advances, it is crucial to address challenges related to bias, inclusivity, and privacy. Overcoming these challenges will unlock the potential of computer vision applications across industries, such as autonomous vehicles, healthcare, and robotics. The future of computer vision looks promising, with innovations in 2D annotation playing a role in shaping it.