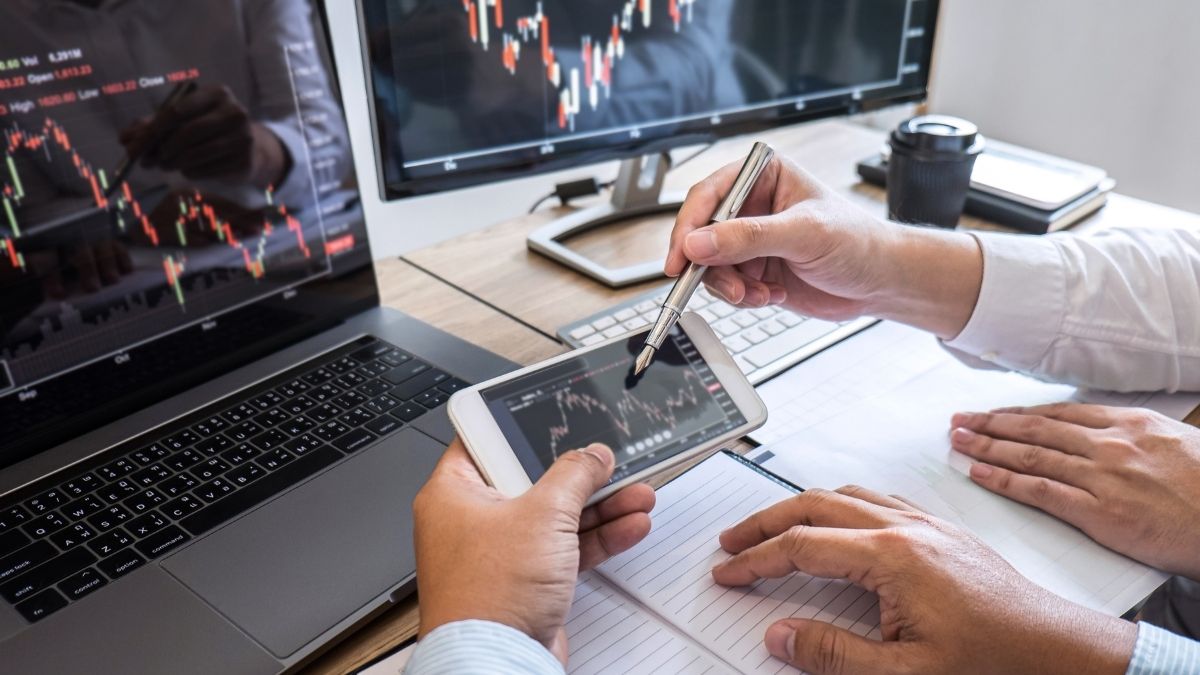
Now that we’ve passed the hype phase of AI, it’s clear that the technology’s biggest problems are focused on making money rather than figuring out how to make it useful. AI is capable of providing immense value for many organizations, thanks to the growing number of AI experts and machine learning services. When it comes to deploying AI, however, companies frequently fail to recoup their initial investments. Isn’t this a little bit contradictory?
According to a recent IBM study, only 21% of businesses are able to integrate AI into their operations. The root of the problem is that it is impossible to achieve economic returns on technology that has not yet been put into production. Furthermore, even when AI projects are deployed, they frequently fail to deliver the expected results.
Let’s talk about the roadblocks that businesses face on their way to making money with AI and how they can be overcome.
Get the workforce ready
Given that AI is always data-driven, the adopting organization’s culture must be data-driven as well. Surprisingly, a lack of data culture is one of the most common issues that businesses face when it comes to realizing AI’s full potential.
AI initiatives will almost certainly fail if the company’s leaders and key employees lack data expertise. Even the most expertly built AI systems will fall short of their full potential if the staff does not use data-driven decision-making. Another common AI implementation mistake is a lack of change management.
Artificial intelligence (AI) frequently necessitates significant changes in organizational structure and strategy, as well as employee mindsets and skills. As a result, think of change management as a critical component of your AI implementation strategy, and make sure your company’s leaders have the knowledge and motivation to foster an AI-centric culture.
Set measurable objectives
While goals are essential for any project’s success, many businesses still struggle to define them when it comes to AI implementation. It’s critical to have clear expectations for an AI initiative’s outcomes. End users rarely participate actively in AI projects, so even if the technical team creates flawless AI systems, they add little value to the business. This is why it’s critical to include all stakeholders in the project from the start.
Furthermore, AI projects frequently provide value that cannot be quantified. Improved employee satisfaction or a better customer experience, for example, are much more difficult to track than cost or time savings. Let’s say you want to build an AI system to help the IT department categorize tickets more quickly. First, because the system will have to make sense of free-form text using natural language processing (NLP), it won’t be perfect at first. As a result, your team will need to calculate the acceptable error rate and factor it into the ROI calculation.
Let’s say there’s a critical issue that requires immediate attention from IT staff, and an AI system incorrectly classifies the ticket as low-priority. This makes calculating ROI much more difficult because it’s difficult to quantify the negative consequences of such a situation.
This is why it’s crucial to start with projects that can be properly calculated in terms of return on investment. Many manufacturing companies, for example, are able to achieve economic returns on AI quality control initiatives because their ROI is relatively simple to calculate.
Begin with a small project
While it’s tempting to build large-scale AI systems, focusing on low-hanging fruit is often a better strategy, especially early on. It might be a good idea to start with robotic process automation (RPA), which is less expensive than AI and has a faster return on investment. RPA is non-invasive, which means it doesn’t disrupt the flow of legacy systems the way many AI solutions do.
Quick wins in AI projects can also help justify more ambitious AI investments and ensure stakeholder buy-in in the future.
AI necessitates maturity
While it may appear insignificant, companies that are more mature and experienced stand a better chance of benefiting from AI. Companies with established data governance practices, elaborate training programs, performance tracking systems, and clear project goals are more likely to be found in this category. These are crucial distinctions between companies that succeed and those that fail when it comes to AI implementation.
Given the erratic nature of project success rates, AI, more than any other technology, necessitates a strong foundation in key management areas. The ability of a company to track, measure, and organize processes is often linked to its likelihood of profiting from AI.
Learn more from Artificial Intelligence and read What Is Artificial Intelligence (AI) and How Does It Work?